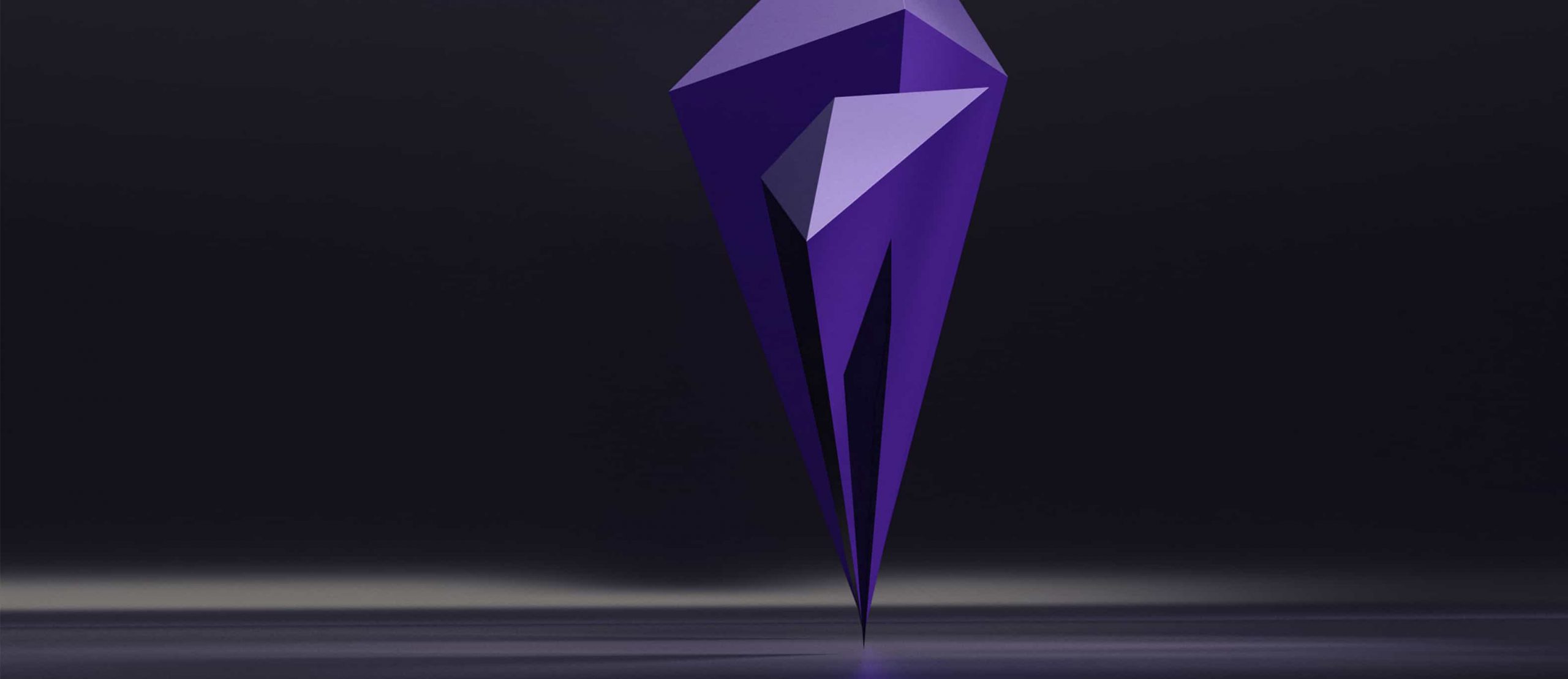
Data and Analytics Trends for 2022
Data and Analytics Trends for 2022
January 25, 2022
Nowadays, it’s becoming increasingly plain to see that data forms the backbone of every organisation out there.
Any business that views data analysis as a core business function is likely to be a well-oiled machine. More and more organisations are starting to unlock the vast potential of data-driven insights, realising how drastically they can streamline business processes and contribute to long-term growth.
As companies come to realise that they are powered by data, it’s critical to be aware of the key data and analytics trends for the year ahead. New technologies are rapidly evolving, whilst existing technologies are advancing at a dramatic pace too. Thus, the landscape of data and analytics is forever shifting. The scope for innovation is huge.
Let’s dive into some of the hot topics in the data and analytics scene for 2022.
Causal AI
According to an October 2020 study, only 10% of organisations see a significant return on investment from their use of artificial intelligence. What new developments could possibly be a solution to this lack of financial return? Enter causal AI.
Causal AI stands out from typical predictive AI by taking the concept one step further. Not only is the technology capable of taking data and predicting future outcomes, but it also identifies the factors that all play a role and have an influence on those outcomes.
Unlike most standard AI systems which often require large pools of data, can crumble under the pressure of novel data, and provide limited explanation relating to decisions, causal AI relays information in a far more comprehensible and reliable way.
Imagination is embedded into causal AI. This means that if there’s a crisis or it comes into contact with unusual data, the technology is able to rationalise critically and respond to unexpected change.
In the era of COVID-19, it’s crucial that AI is able to adapt and respond to novel situations that are a world away from stores of outdated historical data.
Causal AI also presents information in an easily digested way, so that humans can work hand-in-hand with it. It’s helping to democratise data analytics by filtering its findings through a very comprehensible lens. It’s not as difficult to decipher as with most standard AI systems which use black box models.
Explanation is at the forefront of causal AI. It places an emphasis on theĀ why, so that decision-makers have a real understanding of the issues at hand. It creates a link between cause and effect in order to make more accurate predictions. To find out more about this revolutionary technology, take a look at Causalens, a pioneering company when it comes to causal AI.
TinyML
Tiny machine learning is a big innovation that comes in the smallest of packages.
It’s a type of technology that provides on-device analytics on low-powered hardware and software alike. It involves sensors that are capable of detecting and tracking certain sounds, visuals, and movements.
Many companies are introducing this technology in a bid to optimise efficiency, maintain health and safety standards or minimise the negative impact that their organisation has on the environment.
TinyML is able to run on small, battery-powered devices. This saves vast amounts of energy and money by performing tasks on a piece of hardware and reserving cost and power for the processes that truly demand it.
If problems can be identified through TinyML’s on-device sensors, this has the power to improve the efficiency of an organisation. They can hold back and use their more expensive, larger-scale technology where it’s really needed.
This technology is being used more and more, for a number of purposes and spanning a vast array of industries. It’s a far more cost-effective and low-energy method of tracking data in order to monitor a company’s performance.
Generative AI
Generative AI is capable of taking existing data – whether that be in the form of images, text, or sound – and building new content on its own. The technology identifies patterns within pre-existing content and then models its own self-generated content off of it.
People are gradually waking up to the transformative capabilities of generative artificial intelligence. The power it holds is difficult to fathom. Anyone who’s seen a deepfake of a celebrity is aware of how plausible the artificially-produced content can be.
This technology can be utilised in a number of different fields and for a range of purposes. One very common function of generative AI is the processing of images. It’s able to sharpen low-quality pictures, and even restore century-old historical video by creating smooth frame-by-frame transitions and infusing the video with vivid colour, bringing the past to life.
Generative AI is also at the forefront of medical innovation. It can be used in 3D printing as part of the process of creating prosthetic limbs.
It’s even capable of proof-reading text, editing long-format written pieces and, incredibly, composing a narrative out of multiple pieces of information, putting it all together to create an article or story of its own making. It’s awe-inspiring and, to many, truly terrifying.
As exciting and innovative as generative AI is, there are many potential risks associated with it. In the wrong hands, generative AI could be used to scam people or spread misinformation. There definitely is a dark and potentially malicious side to it when used in negative ways.
One thing’s for sure: generative AI is taking the world by storm. It’s sure to develop even further to new and impressive heights in 2022.
The metaverse
A word that is currently on everyone’s lips, the metaverse is a trending topic that is spreading like wildfire. It’s shrouded in mystery, and even though we don’t know much about it yet, it’s hard not to be excited and terrified in equal measure.
As it stands, the metaverse remains a very loosely defined and abstract concept. Broadly speaking, it’s set to be a virtual reality space where the digital and physical worlds collide. It’s going to be designed as a platform for people to work, play, and socialise.
Whilst some envision the metaverse as an entirely virtual space completely removed from the physical world, a kind of world in and of itself, others believe that it will infuse the real world with digital features, creating a kind of augmented reality.
Many mega corporations are gearing up to be major players in the metaverse, including tech giants Microsoft and Facebook (who recently rebranded themselves, rather fittingly, as ‘Meta’).
The metaverse is still in the early stages of development, very much a fledgling project. Because of this, the building of the metaverse will depend heavily on real-time data analytics. Much of the content on the platform will be user-generated, and it will be incredibly interesting to see how this gradually unfolds in practice.
AutoML
Automated machine learning has already been adopted by many companies, who are beginning to reap the benefits of this technology. AutoML is growing in popularity, and it’s easy to see why.
AutoML is the automation of data discovery, facilitating the way in which we gather insights. It’s a key development in the data world, that has dramatically changed the way in which data scientists work.
Formerly tasked with manually preparing data and inquiring into model hyperparameters due to the fact that they were the ones with the niche machine learning knowledge and expertise to carry this out, the data scientist is now free to turn their attention away from less dull and tedious tasks and towards more engaging and impactful work.
Thanks to this technological advance, the data analyst is now at liberty to focus on more value-add tasks. AutoML is by no means capable of replacing human expertise, it simply means that the data analyst is no longer bound to technicalities.
In the past, machine learning was beyond the remit of non-ML experts, but AutoML has changed the game in this respect, opening up the world of machine learning and contributing to the democratisation of data. This is a progressive change that makes machine learning more accessible to ‘citizen’ data scientists.
Chances are, AutoML will become a fundamental business practice for many companies in 2022.
Natural language processing
Whilst natural language processing is by no means new technology, it’s developing at a rapid rate and will have a huge impact in years to come. We’ve only scratched the surface of NLP’s capabilities.
Computers typically do not speak our language – they use machine code, a secret language known by a select few. That is, until NLP came along. Natural language processing makes human-machine communication far easier, as it allows us to communicate with computers using our own language. It’s breaking down the wall between machines and humans, by rendering machines capable of understanding voice and text data and responding through the same medium, using human language.
For those who do not have coding experience or computer science backgrounds, this has the potential to be revolutionary. The technology is still not perfect, and uptake is still relatively low. However, we’re convinced that it could dramatically contribute to the democratisation of data in coming years. People without coding abilities can use NLP to make data queries and reach insights through written and spoken word.
In many real-life contexts, NLP is already an established part of everyday reality. Whether it’s through your voice-operated GPS, online customer service chatbots, translation tools such as Google Translate or virtual agents like Amazon’s Alexa, you’ve probably interacted with NLP in some capacity before.
But the role of NLP is expanding as businesses begin to adopt it as a means of streamlining business processes. Many companies are starting to utilise NLP in order to gather data from emails, surveys, customer calls and social media in order to gauge customer sentiment. It offers a new and innovative way of measuring company performance and customer behaviours. Customer feedback can be reviewed via NLP in order to analyse the collective opinion of the company’s audience.
Demand for data storytellers
With this influx of data innovation comes an increase in demand for analysts with the ability to transform data into actionable insights. Data volumes are growing, and many businesses now view data analysis as a core business function rather than a distant, separate department occupied by a few specialists.
The data job market is booming. After COVID-19 first hit, a LinkedIn report found that of the top five fastest-growing skills, two were directly related to data and analytics.
If there’s one thing in particular that is highly sought after at the moment, it’s data visualisation skills. Although AI and ML technology allow you to glean insights from data with more ease, the volume of data is rapidly growing. And thus, there is a high demand for users who can tell a story with that data, creating a cohesive and accessible narrative by carefully selecting the insights that are meaningful to the business.
Data storytelling allows users to paint the bigger picture and communicate that to the executive level of the company, acting as a mediator between the data scientist and the wider business. To succeed at this, a deep understanding of the business and the way it operates is crucial, as is the ability to communicate concisely and effectively. Niche expertise of coding and computer science is no longer the be-all and end-all.
With the democratisation of data, insights are no longer in the hands of data specialists alone. Data analysis is a core business function that companies are coming to rely more and more upon to drive success.
If you’re thinking of hiring data scientists, data engineers or analysts into your business in 2022 and would like some help, please get in touch with us at contact@nicholsonglover.co.uk or visit our website for more information.